Dr. Shu-Fen Wung's Newly Funded Research Uses Technology to Study Falls in Older Adults
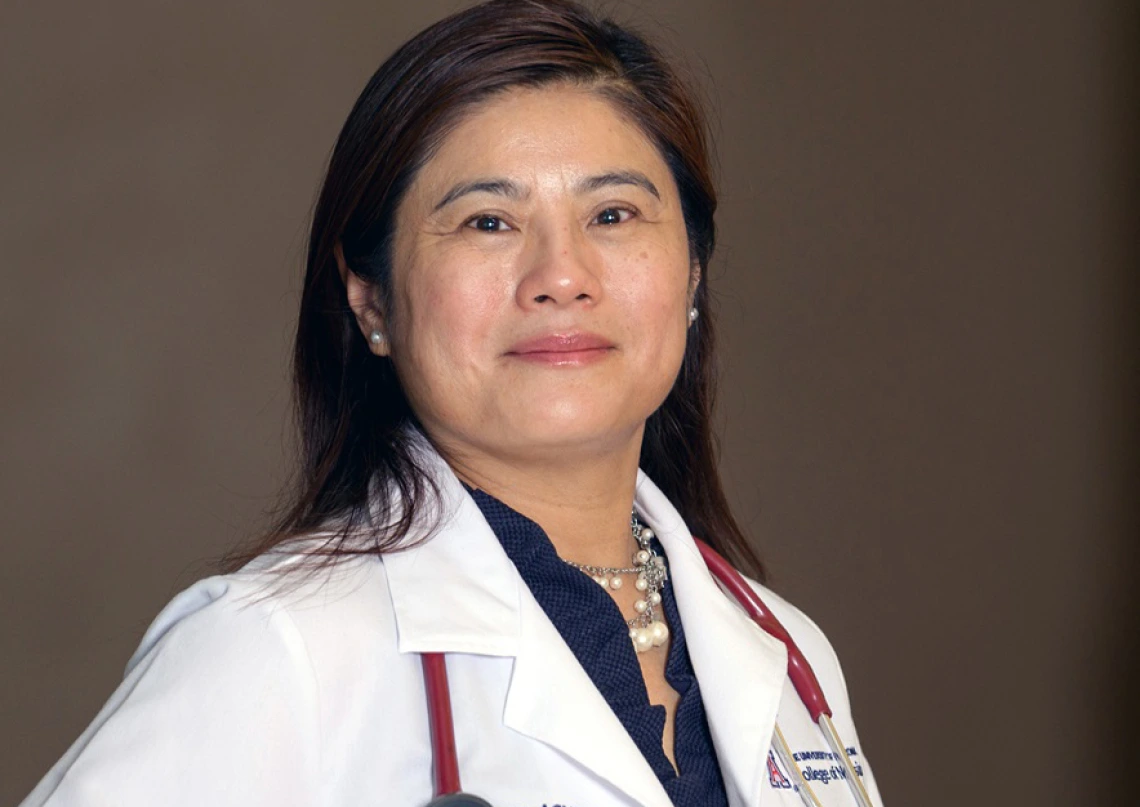
Shu-Fen Wung , PhD, MS, RN, ACNP-BC, FAAN
The University of Arizona College of Nursing is proud to acknowledge the achievement of Associate Professor Shu-Fen Wung , PhD, MS, RN, ACNP-BC, FAAN, for receiving funding for her innovative new study, "Using Contactless Sensors to Remotely Monitor Falling." Dr. Wung and Dr. Marjorie Skubic from University of Missouri College of Engineering will conduct a study to evaluate the potential to use contact-free passive sensing to continuously monitor older adults for falls.
"Falling is the second leading cause of death from unintentional injuries worldwide," Dr. Wung said. "The greatest number of fatal falls occurs in adults older than 60 years of age. Approximately 20-30% of older adults who fall suffer moderate or severe injuries, such as hip fractures and head injuries. Older adults who fall and suffer a disability are at a major risk for subsequent long-term care and institutionalization. Studies have shown that older adults are very concerned about falling and felt helpless and fearful if falls are not detected in a timely manner. Although various existing studies focus on fall detection with individual sensors, such as wearable ones and depth cameras, the performance of these systems is still not satisfying. In this project, we are collaborating with Dr. Alisha Johnson from the University of Missouri College of Nursing, Dr. Janet Roveda from the University of Arizona College of Engineering, Signify (formerly known as Philips Lighting), Best Buy Health, and assisted living facilities (Via Elegante Tucson Mountains and TigerPlace) to use innovative ambiance-based light-embedded radar sensors to provide a robust and sensible technological solution to remotely monitor falls in real-world settings. With advances in sensor technology and machine learning, this project will revolutionize the way falls are detected in older adults."
MPI: Shu-Fen Wung and Marjorie Skubic
Title: Using Contactless Sensors to Remotely Monitor Falling
Funder: NSF IUCRC Center to Stream Health in Place (C2SHIP)
Award Period: 7/1/23-12/31/23
Description: This study will utilize light-embedded nonwearable technologies to 1) test the developed algorithm for the false positive (FP) and false negative (FN) rates of fall detection with remotely patient monitoring, and 2) collect the data regarding gender, age, health condition etc. to improve the algorithm performance and build a trustworthy AI system. A secondary purpose is to 3) validate a wearables-based continuous fall risk estimation algorithm, using the study’s ground truth sensors.
Assisted Living Facilities: Via Elegante Tucson Mountains, and TigerPlace